2022 AI Predictions: Metaverse Up Ahead, Consumer Privacy Push, Data Fabrics Coming
More 2022 AI Predictions: agile data teams, data equity focus, another algorithmic business failure, industrial data scientists coming; rise of your personal digital avatar
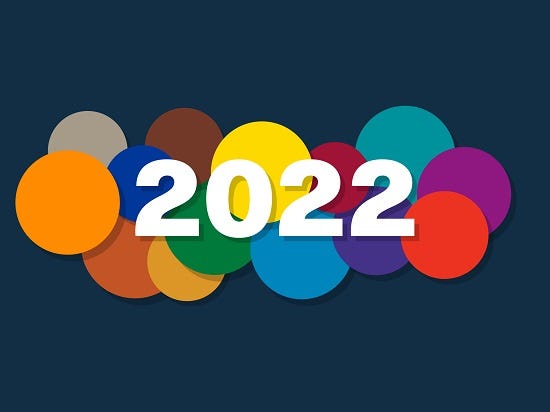
[Ed. Note: We have heard from a range of AI practitioners with predictions for the impact of AI in 2022. Here is a selection:]
From Dr. Lance Eliot, Chief AI Scientist at Techbrium Inc. and a Stanford Fellow at Stanford University, offers his Top 5 predictions about the state of AI to emerge in 2022:
Metaverse Roars Ahead Via AI: The metaverse kicks into high gear in 2022 as AI enables virtual world capabilities that draw into the nether realm a slew of new business opportunities. Real-world businesses will rapidly set up AI-driven online entities and eager consumers will arrive in droves to see what it is all about.
AI Inside Goes All In: You can expect more and more products to have embedded AI that will transform goods into being smartish, enabling immediate customization to meet customer needs. The hallmark will be that the so-called AI inside is the business imperative for 2022.
Ethics And AI Intertwine Seriously: Though the push toward Ethical AI will continue stridently in 2022, regrettably it will be an uphill battle. There are going to be some big-time AI ethical bombshells that will rock the AI headstrong efforts, prompting the insidious AI For Bad to briefly overshadow the AI For Good upbeat movement.
Drive-Thru AI Takes Your Order: Do not be surprised in 2022 that when you use your local drive-thru there is merely a disembodied computer-based voice that takes your order. Yes, conversational AI will be working the order desk as you drive up to a to-go eatery display panel and place your food order. People will be perfectly fine with this due to increased ordering accuracy and faster processing speed.
AI Legal Personhood Still A No Go: You will undoubtedly hear unbelievably amazing stories in the news that AI is going to be anointed with legal personhood, meaning that AI will be considered akin to humans and hold similar constitutional rights and privileges. Hogwash, this is pure nonsense, and there isn’t any AI in 2022 that can genuinely be considered anywhere near to personhood (those unbelievable stories are in fact not to be believed).
(Dr. Eliot is a prolific author on AI, with his popular columns on AI having amassed over 4.5 million views and his podcasts downloaded over 200,000 times. He can be followed on Twitter at @LanceEliot.)
From Dr. Max Versace, PhD, CEO and co-founder of Neurala:
AI will migrate from digital to physical applications. In 2020 and continuing into 2021, the world has been awakened to real, physical problems. As a result, the focus of AI applications will shift from digital domains to physical ones, where AI can play a pivotal role in helping us solve real-world challenges. For example, AI applications that shape our physical world –the ones that remove key vulnerabilities in manufacturing, supply chain, and logistics – will take the spotlight. AI will come of age and enter adulthood. One example of a physical function demanding AI is quality inspections, a task traditionally performed by human workers. AI’s physical impact could be huge for the 35 million workers - roughly the population of Canada - devoted to performing this basic function on the manufacturing floor. The year 2022 will be a pivotal moment for AI: the urgency many manufacturers face needs innovative technology to help cope with pandemic disruptions to our physical economic infrastructure.
AI will become ubiquitous by becoming hyper-accessible. Up until now, AI applications have been mostly in the hands of experts. Only recently have more Auto Machine Learning (AutoML) platforms appeared on the market. Created by both startups and larger industry players, these platforms seek to address the scarcity of AI talent. It’s estimated 30 percent of enterprises plan to incorporate AI into their company within the next few years, with 91 percent foreseeing significant barriers and roadblocks for AI adoption. With only a fraction of these companies having AI-enabled or fluent personnel, AutoML platforms that enable non-experts to quickly build and deploy applications will be key. In manufacturing for example, AI platforms will provide integration hooks, hardware flexibility, ease of use by non-experts, the ability to work with little data, and, crucially, a low-cost entry point to make this technology viable for a broad set of customers.
From Varun Ganapathi, Ph.D. (AI from Stanford), cofounder and CTO of AKASA, offering AI for revenue cycle management:
Companies will lean more on human-powered AI to avoid “Garbage In, Garbage Out” algorithms. As AI continues to evolve at a breakneck pace, companies often overlook the importance of keeping humans actively involved in the AI implementation process, creating a scenario where tech’s obsession with the newest, biggest thing neglects basics that make AI actually useful. That is, plugging in useful data and teaching it how to deal with outliers.
For AI to truly be useful and effective, a human needs to be present to help push the work to the finish line. Without guidance, AI can’t be expected to succeed and achieve optimal productivity. This is a trend that will only continue to increase. Ultimately, people will have machines
report to them. In this world, humans will be the managers of staff (both other humans and AIs) that will need to be taught and trained to be able to do the needed tasks.
Just like people, AI needs to constantly be learning to improve performance. A common misconception is that AI can be deployed and left unsupervised to do its work, without considering the reality that our environments are always shifting and evolving.
Machine learning and human-in-the-loop approaches to automation will displace RPA.
Digital transformation efforts in a number of industries have driven massive adoption of robotic process automation (RPA) during the past decade. The hard truth is that RPA is a decades-old technology that is brittle and has real limits to its capabilities - leaving a trail of broken
bots, which can be expensive and time-consuming to fix.
RPA will always have some value in automating work that is simple, discrete, and linear. However, automation efforts often fall short of aspirations because so much of life is complex and constantly evolving - too much work falls outside the capabilities of RPA.
Why the AI community needs to go back to basics - data labeling. Solid AI systems rely on two things: a functioning model and underlying data to train that model. To build good AI,
programmers need to spend collecting, categorizing, and cleaning data. The gut instinct of many AI technologists is to run towards the sexy work of creating a complex AI infrastructure and neglect the basics of data labeling. We need to go back to basics in order to make AI work at its true potential.
From Shubham A. Mishra, CEO and Co-Founder of Pyxis One, a codeless AI infrastructure tech startup, which recently raised $17M in funding:
A Cookieless World Wide Web is closing in on us more rapidly than ever before. In the complete absence of cookies (as impossible as that may seem), marketers will need to depend on other ways to understand consumer behavior, target accurately and optimize efficiently. So, developing tools and tech to help marketers can shape up to be a hot space.
Democratization of AI. Adoption of no-code, low-code AI may prove to be the biggest strength for marketers and advertisers against the backdrop of all the privacy and cookieless web debates. Giving advertisers effortless, simple and quick access to tools that can aggregate first as well as third party data, will allow them to nimbly navigate publisher and platform-specific restrictions.
Apple’s App Tracking Transparency update may just be the starting point for the privacy wars between major publishers. Apple also seems to have a plan in this area and is executing in stages. The biggest stories are likely to revolve around privacy concerns of consumers, new technology, data usage and advancements in the virtual work space.
Data Accountability. With all the news about protecting, and being transparent about consumer privacy, it only makes sense for consumers to want more clarity on how their data is being used. More information on what consumers are saying yes to when they click on the “I Agree” button of privacy policies is a huge potential story.
VR & AR is the next big thing. VR & AR is the next big thing. We are witnessing an increased pace of acquisitions of VR & AR startups, so it's going to be interesting to see if, and how, brands incorporate AR into their marketing strategy.
Natalie Monbiot, Head of Strategy, HourOne, providing AI-based video production using synthetic characters:
In 2022 look for the continued rise of the digital human as businesses and their people look to capitalize on their digital selves to drastically improve communications, save time, money and resources.
2022 will see the growth of a new hybrid workforce in which human employees share their workload with digital employees. They will offload repetitive or routine tasks to machines that can perform them just as well, and in some cases better.
What’s more, employees will have their own digital avatars, with superhuman skills - such as the ability to speak any language. This will serve to break down geographical and cultural barriers and enable a whole new era of frictionless communications.
The new augmented, hybrid workforce will become pervasive thanks to advances
in AI video production, and the sheer portability of video. This will play well with asynchronous communication, which is proven to be the most effective medium for the remote working environment.”
From Jared Peterson, Senior Vice President of Engineering, SAS, multinational supplier of analytics software:
COVID changed (and will continue to change) machine learning algorithms. The pandemic upended expected business trajectories and exposed the weaknesses in machine learning systems dependent on large amounts of representative historical data, including well-bounded and reasonably predictable patterns. As a result, there is a business need to reinforce the analytics “core” and bolster investments in traditional analytics teams and techniques better suited to rapid data discovery and hypothesizing. Synthetic data generation will play a major role in ensuring the availability of sufficient representative data in the dynamic environments witnessed today.
AI and machine learning specialties expand at a staggering pace. Advances in deep learning, the computing power necessary to enable those advancements (e.g., GPUs), and the frameworks that make it all accessible have brought about a renaissance in the world of computer vision and NLP. We will also see highly publicized, human-level and beyond performance, enabled by areas like reinforcement learning. As the pace of research and innovation continues to grow, we will see advancements in one area influence another area (e.g., transformer architectures moving from NLP to computer vision).
From Tapan Patel, AI & Analytics Senior Manager, SAS:
Data fabrics reign in big data. Amid the growing complexity of data, organizations will continue to struggle to deliver connected and consistent data across on-premises, public and multi-cloud sources. They’ll need to do this while supporting different use cases or different types of users that need trusted, consistent and real-time data to build apps or deliver insights. To reign in big data, data fabrics must focus on ingesting, transforming and cleansing data through pipelines in a governed manner. Part of this process means automating certain processes and steps in the DataOps cycle, and securely pushing down logic and processing to various data platforms.
AI matures past model development. Gone are the days of investing in building the perfect model. There is a lot more maturity when it comes to approaching productive deployments for AI across industries. The focus will shift to the broader ecosystem needed to deliver AI projects, and organizations will realize enhanced value by investing in data management capabilities and deploying and governing AI and analytical assets.
From Andrew Kasarskis, Chief Data Officer at Sema4, working on AI in precision medicine:
Efficient allocation of data curation resources: We need technical and process innovation around the collection of biomedical data. When obtaining the large corpuses of well-labeled data to train the AI, some human manual and semi-manual work is inevitably needed. This work is always expensive, never scales well, and frequently takes experts with esoteric knowledge away from important value-generating activities. Figuring out the most efficient way to allocate manual curation work is a significant unmet need that impedes progress in the use of data technology, particularly in biomedicine.
Continued focus on data equity: Societal biases and inequities can be present whenever data is used. I expect individuals and organizations to continue discovering errors, omissions, and blunders in their data where biases in collection and storage led to incorrect, misleading, and harmful outcomes. Continued focus on identifying and resolving these issues is important for both accuracy of conclusions and equity in data use.”
From Nick Elprin, CEO, Domino Data Lab, supporting data scientists:
The Chief Analytics Officer will eclipse the Chief Data Officer. While many
companies today have a chief data officer, in 2022 we will see more enterprises establish “chief analytics officer” or “chief data and analytics officer” roles. Elevating analytics reflects an evolving understanding of data science and machine learning as the ultimate functions that turn data into business value, and increasingly core to company-wide strategy.
Democratization of ML through upskilling will make more analysts comfortable with code. For over 20 years, different products have promised to enable advanced analytics with “no code” or “drag and drop” user interfaces. The latest wave of this trend will lose enthusiasm in favor of companies investing to upskill their workforce. Analytical programming languages
like Python and R will become more table stakes (especially with the rise of data science degree programs in secondary education), just as Excel and SQL became a decade ago.
We’ll see another major public failure of an algorithmic business. While there’s no public post-mortem of Zillow’s dramatic exit from the iBuying market, Zillow is a warning sign about the risks of algorithmic business. Model-driven businesses are immensely powerful, but also hard to get right. As more companies develop model-driven strategies, we will see more of them stumble — either because they didn’t properly manage probabilistic risks, didn’t properly integrate data science with business processes and domain knowledge, or relied on too much “magic” without understanding fundamentals and statistics.
Unpredictable business conditions will accelerate adoption of model monitoring. Model monitoring, already critical in a post-pandemic economy, will become essential. The continued volatility of unpredictable business factors, from supply chains to extreme weather, will greatly accelerate the need for businesses to continuously monitor how well their models
reflect the real and rapidly changing world of their customers.
Reza Morakabati, Chief Information Officer, Commvault, offering data protection and data management services:
Enterprises Bring DevOps’ Agility to Data Management . Over the next year, enterprises will launch new agile, DevOps-like teams dedicated to optimizing how they manage and use the enterprises’ data. These groups -- composed of data security, protection, analytics and other types of data experts, along with IT operations staff – will be tasked with quickly and efficiently improving the security, protection, governance, and value of their organization’s data.
These DevOps-like agile data teams are needed because, having accelerated their digital transformation initiatives during the pandemic, enterprises now find themselves unable to view or control all the data they have sprawled across dozens of SaaS applications, multiple cloud services, and various types of on-premises infrastructure. They also need agile data teams that shorten cycle times for new data management solution releases. This will increase the frequency of these releases so that IT and business teams can experiment with them and quickly provide feedback on how well they help improve business outcomes.
Agile data teams can gain centralized visibility and control, allowing them to secure data against attacks from cybercriminals, recover data of a cyberattack is successful, and move data between SaaS applications, clouds and infrastructures if necessary. These teams can also work with business line employees to find new ways to use data analytics, AI, ML and other tools to glean valuable insights for this data that improve their business outcomes.
Those enterprises that create agile data teams next year will be able to continuously optimize how they store, protect, secure, govern, and use their data – allowing them to ensure the fundamental integrity of their business while also liberating them to do amazing things with this data.
From Bill Scudder, SVP, AIoT General Manager, AspenTech, providing asset optimization software and services for process industries:
The generational churn occurring in the industrial workforce will inspire the widespread emergence of industrial data scientists as central figures in adopting and managing new technologies, like industrial AI, and just as importantly, the strategies for deploying and maximizing these technologies to their full potential. New research has revealed that while 84 percent of key industrial decision makers accepted the need for an industrial AI strategy to drive a competitive advantage – and 98 percent acknowledged how failing to have one could present challenges to their business – only 35 percent had actually deployed such a strategy so far. With one foot in traditional data science and the other in unique domain expertise, industrial data scientists will serve a critical role in being the ones to drive the creation and deployment of an industrial AI strategy.
2022 will also see AI’s maturation into industrial AI reach full bloom, graduating to real-world product deployments with concrete time-to-value. To achieve this, we’ll see more industrial organizations make a conscious shift from investments in generic AI models to more fit-for-purpose, precise industrial AI applications that help them achieve their profitability and sustainability goals. This means moving away from AI models that are trained on large volumes of plant data that can’t cover the full range of potential operations, to more specific industrial AI models that leverage domain expertise for interpreting and predicting with deep analytics and machine learning. Industrial data will be transformed into real business outcomes across the full asset lifecycle.
This shift will have the dual benefit of also facilitating new best-of-breed alliances built around industrial AI. Previously, partnerships were very tech-centric, driven by services or one large vendor. The more specialized focus of industrial AI will require a larger set of solutions providers, pooling together their independent and customized expertise. Not only does this help evolve partnerships away from more generic AI projects, it will also place a greater focus on time-to-value partnerships as opposed to do-it-yourself approaches, helping to lower the barrier to AI adoption more than ever.