Data is the New Focus of Seriously Ethical AI
The BigScience global effort by 1,000 researchers in 60 countries is building a transparent, accountable large-language model called BLOOM. The French government is helping to fund it.
By John P. Desmond, Editor, AI in Busines
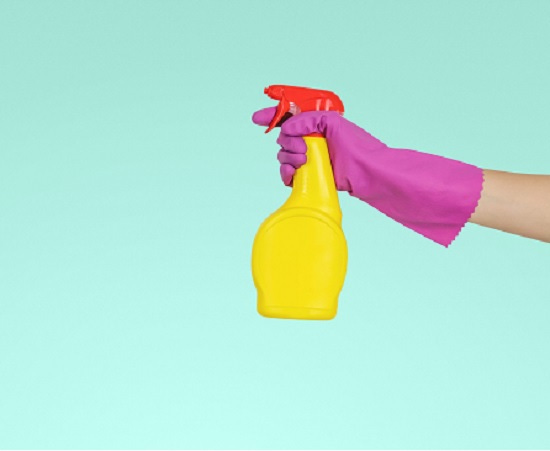
Some data scientists are uniting in an effort to work with better quality data to build AI systems.
BigScience is a global effort by 1,000 researchers in 60 countries to build a more transparent and accountable AI, with less of the bias that has hurt many AI projects. Primarily volunteers, the participants trained an AI system with good data curated by humans from different cultures, as opposed to with data scraped from the internet, written primarily in English and polluted with hateful speech on race, gender and religion. The system was released on July 12 for researchers to study.
The model can be accessed at Hugging Face, the site of an AI community working to democratize AI.
“The industry folks don’t really care about the data. They just grab whatever’s easiest,” stated Maarten Sap, a natural language processing researcher, in a recent account in The Washington Post. “People think it’s all the same, and you just need more of it,” stated Sap, who will begin work as a professor at Carnegie Mellon’s Language Technologies Institute this fall.
The Big Science project is focused on researching large language models, the same research subject that has resulted in multiple ethics engineers being fired from Google.
The data chair for the BigScience project, Yacine Jernite, led a team that recruited communities of native speakers, beginning with eight commonly spoken languages including Arabic, Chinese and Spanish. More than 60 percent of the 341 billion-word data set used to train the AI model, called BLOOM, was handpicked by the team.
Jernite, a child of Moroccan parents, works for Hugging Face, the open source AI startup. Big Science has received a grant from the French government to use the Jean Zay supercomputer outside Paris to conduct its research. Jernite stated in the Post that this helps the team avoid the “choices of convenience” that may handicap projects seeking to reduce data bias.
More Languages In BLOOM Model Any Any Other LLM
If the team’s experience is any indication, efforts to produce unbiased data sources to train AI systems will break along cultural and ethnic lines. BigScience sought to involve communities around native speakers from the start, asking them to provide data reflecting their culture. The groups included Masakhane, an African machine learning group, LatinX in AI, Machine Learning Tokyo, and VietAI.
Specialists are even emerging within languages, to help with regional dialects. One example is Maraim Masoud, an ML engineer originally from Libya and now based in Europe, who is focused on Arabic. She and her colleagues expanded their work for BigScience into Masader, a catalog of Arabic data sets, according to the Post report. Most datasets focus on standard Arabic, used for example in newspapers. Fewer datasets exist on Arab dialects, which are typically used in social media and can differ greatly from standard Arabic.
She is now working to evaluate the BigScience Model on bias and toxicity. She is hopeful. “Even with GPT-3 [OpenAI large language model], the intention was not to have a biased model,” she stated. “Humans are testing it and as they do, it will reveal a lot of shortcomings and wrongs. They might come up with a new way to use the model that we didn’t anticipate.”
BLOOM, which stands for BigScience Large Open-science Open-access Multilingual Language Model) is designed to be as transparent as possible, according to a recent account in MIT Technology Review. The researchers have shared details about the data it was trained on, the challenges in its development and the way its performance is evaluated. In contrast, OpenAI and Google with its LaMDA project, have not shared their code or made their models available to the public, so little is known about how their models are trained.
At 176 billion parameters that determine how input data is transformed into the desired output, B:LOOM is bigger than OpenAI’s 175-billion-parameter GPT-3. BigScience maintains that it offers similar levels of accuracy. For Spanish and Arabic, BLOOM is the first large language model of this size.
Large language models are expensive to develop, putting their development out of reach of many in the world. BLOOM benefits from the support of the French government. The BigScience team is embedding ethical considerations into its model from inception. The group has developed data governance structures to make more clear what data is being used and who it belongs to.
BigScience Team Proposes a Responsible AI License
The group is also launching a Responsible AI License, like a terms-of-service agreement, designed to deter the use of BLOOM in high-risk sectors such as law enforcement and health care, or to harm or deceive people. Its co-creator, Danish Contractor, describes it as an experiment in self-regulating LLMs. Contractor is a senior researcher at IBM responsible for AI licensing, based in the New York City area.
The English language dominates LLM research, but BLOOM is different. It can understand 46 languages, including 13 Indic languages, such as Hindi, and 20 African languages, according to the MIT Tech Report account. Just over 30 percent of its training data has been in English; the model can also understand 13 programming languages.
To achieve this diversity in its training data, the team engaged in efforts such as organizing workshops with African AI researchers, according to Chris Emezue, a researcher at Masakhane, an organization focused on including African languages in natural language processing (NLP) research.
“If you want to include African languages in the future of [natural-language processing] … it’s a very good and important step to include them while training language models,” stated Emezue.
BigScience is credited with helping to build a transparent community around its BLOOM LLM and for incorporating ethics and governance from the outset, stated Percy Liang, director of the Center for Research on Foundation Models at Stanford University. However, little has changed in overall LLM development. “OpenAI and Google and Microsoft are still blazing ahead,” Liang stated.
The Hugging Face team includes a second-generation AI ethicist in Margaret Mitchell, who is chief ethics scientist at the company, and who was formerly a staff research scientist at Google who led an AI ethics team. She separated from Google over disagreements about how LLM research was being conducted, differences that also caused the separation of AI ethicist Timnit Gebru from Google, and later AI software engineer Blake Lemoine from Google. (See AI in Business, June 27, 2022)
Mitchell sees advantages in the ability of researchers to interrogate the strengths and researchers of the BLOOM model, the MTR account said.
Improved Data Underlying AI An Academic Subject at Stanford
Improving the quality of data underlying used to train AI models is also a subject of academic study. For example, at Stanford University, James Zou, assistant professor of biomedical data science, and a member of the Stanford Institute for Human-Centered Artificial Intelligence (HAI), is emphasizing the data side of AI.
“One of the best ways to improve algorithms’ trustworthiness is to improve the data that goes into training and evaluating the algorithm,” he stated in a press release issued by HAI. Zou and other researchers from ETH Zurich, a public research university in Zurich, Switzerland, recently conducted a two-day Data-Centric AI Virtual Workshop.
“Creating good datasets for AI models has been an artisanal process,” Zou stated. “The goal of the workshop was to explore how to turn that process from an art into a more principled scientific and engineering discipline.”
Themes for the workshop included the importance of shifting from a model-centric to a data-centric perspective, the need to develop benchmarks for each step of the data pipeline, and the value of getting more communities involved in building datasets for AI.
“As AI model-building rapidly matures,” Zou stated, “most of AI researchers’ time and resources will need to be devoted to these data issues.”
Read the source articles and information in The Washington Post, in MIT Technology Review and in a press release issued by the Stanford Institute for Human-Centered Artificial Intelligence (HAI).
(Write to the editor here.)