Explainable AI: A Work in Progress
Experience is low with tools, techniques for making AI explainable, with a skew so far toward helping engineers and mostly neglecting how models impact society
By John P. Desmond, Editor, AI in Business
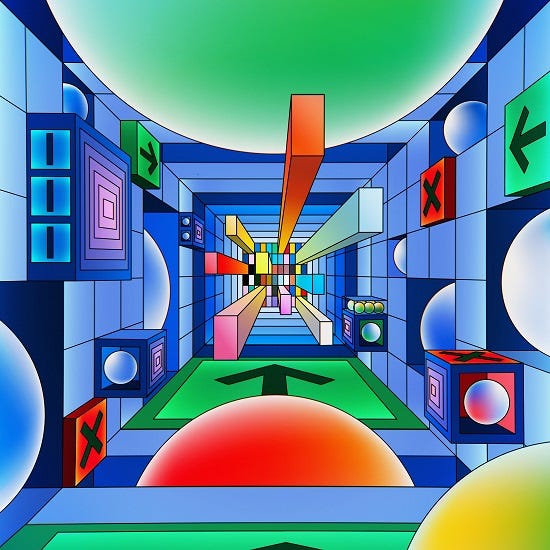
The reasons needed to explain how an AI system came to its decision or recommendation, are many: A student is not accepted by the target university; a production line worker is concerned that a new AI-generated set of instructions is not safe; a doctor’s recommendation does not seem consistent with the patient’s own experience; or shoppers on Black Friday are not meeting expectations of the retailer.
The fundamental idea of explainable AI (XAI)--to provide transparency about how an AI model arrived at its decision–has long been sought in the field. So what is the status of XAI?
A recent blog post from Algolia, a company offering a personalized search engine using AI/ML, provides insight. “So business and government, not to mention individual consumers, are in agreement that AI decision-making must clearly convey how it’s reached a decision. Unfortunately, that’s not necessarily achievable all the time due to the complexity of the AI process, with subsequent decisions being made on the backs of initial decisions,” stated Vincent Caruana, Senior SEO Web Digital Marketing Manager for Algolia, author of the account
.The current state of AI explainability is not good enough, and will make it difficult for users to trust AI, he suggests. “There must be AI-process explanations. People’s comfort levels, companies’ reputations, even humanity’s ultimate survival could well depend on this,” Caruana stated. “In short, businesspeople, their customers and partners, and oversight agencies must all be able to audit and comprehend every aspect of the AI decision-making process.
This notion was seconded by a researcher at the Software Engineering Institute of Carnegie Mellon University in a recent blog post. “AI researchers have identified XAI as a necessary feature of trustworthy AI,” stated Violet Turri, assistant software developer in SEI’s AI Division, author of the post.
Defining XAI for a Range of Stakeholders
Turri defines XAI as a “set of processes and methods that allows human users to comprehend and trust the results and output created by machine learning algorithms,” a definition meant to cover a range of audiences. Researchers in healthcare, for example, see explainability as a requirement for AI clinical decision support systems; in finance, explanations are needed to comply with regulations and help analysts make decisions.
“Explainability aims to answer stakeholder questions about the decision-making processes of AI systems,” Turri stated.
The form of explanations varies greatly based on context and intent, she noted. Researchers are experimenting with tools, including TensorFlow Playground, which helps ML scientists visualize the layers of a neural network, and to watch as neurons change through training. For example, “Heat-map explanations of underlying ML model structures can provide ML practitioners with important information about the inner-workings of opaque models,” Turri stated.
Explainable AI now has its own stages in the ML lifecycle, as Turri described, including: ‘pre-modeling,’ methods for analyzing data used to develop modeling; ‘explainable modeling,’ which interprets the system architecture; and ‘post-modeling,’ to produce post-hoc explanations of system behavior.
The pursuit of XAI has been shown to have benefits. A study by IBM Turri quoted found that users of its XAI platform achieved a 15 to 30 percent improvement in model accuracy, and increased profits.
Constraints facing use of XAI include a lack of experience. Turri stated, “real-world guidance on how to select, implement, and test these explanations to support project needs is scarce. Explanations have been shown to improve understanding of ML systems for many audiences, but their ability to build trust among non-AI experts has been debated.”
In response to requirements of US federal agencies including the Department of Defense, SEI is assembling XAI resources, including 54 examples of open-source interactive AI tools from academia and industry. The findings will be published in a future blog post.
Darpa Has Been Working on XAI Since 2016
The US Defense Advanced Research Projects Agency (Darpa) has been working on XAI since 2016; it has been an elusive quest. “After years of research and application, the XAI field has generally struggled to realize the goals of understandable, trustworthy, and controllable AI in practice,” stated Jessica Newman, co-director, UC Berkeley AI Policy Hub, in a 2021 account for Brookings.
Studies have shown that the gap between results and expectations has stemmed from engineering priorities being placed above other considerations, so that explainability then does not meet the needs of users, external stakeholders and communities affected by the AI system. Choices need to be explicit about what is being optimized and why, Newman suggested.
“The end goal of explainability depends on the stakeholder and the domain,” Newman stated, noting, “Achieving explainability goals in one domain will often not satisfy the goals of another.”
The inner workings of AI systems that affect the lives and opportunities of people, have been behind the scenes, in what Newman called an “asymmetry of knowledge,” that increases the power differential between those creating AI systems and those who bear their impact.
She described this as “one of the key dilemmas at the heart of explainability.”
In practice, the reality of how explainability methods are being used in organizations “diverges sharply from the aspirations,” according to a 2020 study of explainable AI deployments cited by Newman. Most of the deployments were used internally to support engineering efforts, rather than reinforcing trust with users or external stakeholders.
Interviews were conducted with some 30 people from profit and nonprofit groups deploying elements of XAI in their operations. Global explainability techniques, which aim to understand the reasoning used by a model, “were described as much harder to implement,” Newman stated. One reason was a lack of clarity about the objectives.
Overall, the studies showed that early XAI efforts have best represented certain engineering objectives, while the goals of “supporting user understanding and insight about broader societal impacts, are currently neglected,” Newman stated.
She offered five recommendations as a roadmap for moving forward:
Diversify XAI objectives;
Establish XAI metrics;
Mitigate risks;
Prioritize user needs; and
Explainability isn’t enough
On the last point, she stated, “Simply having a better understanding of how a biased AI model arrived at a result will do little to achieve trust.” More trust will come from problems being mitigated, she suggested.
Forebodingly, she stated, “Without clear articulation of the objectives of explainability from different communities, AI is more likely to serve the interests of the powerful.”
Read the source articles and information in a blog post from Algolia, a blog post from the SEI of CMU, and from Brookings.
(Write to the editor here.)