Getting a Read on the Carbon Footprint of AI Development
Early studies show AI developers have options for reducing AI’s carbon footprint; some AI conferences now require info on CO2 emissions to be submitted with papers
By John P. Desmond, Editor, AI in Business
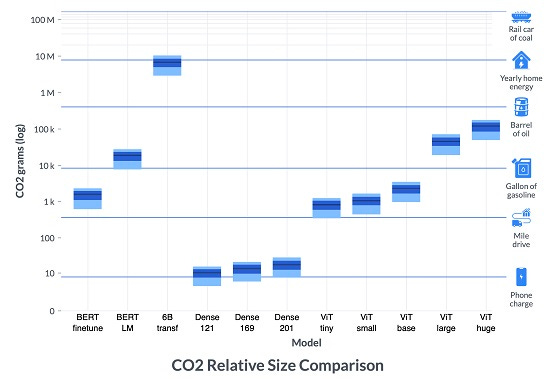
To train large language models, the biggest tech companies are using enormous amounts of energy. It’s not easy to quantify how much energy this is, but efforts are being made to do so.
Sasha Luccioni, a researcher at AI company Hugging Face, tried to quantify the carbon impact of her company’s large language model called BLOOM, an alternative to GPT-3 from OpenAI. And based on a limited set of available data, she tried to estimate the carbon impact of OpenAI’s ChatGPT LLM.
“We’re talking about ChatGPT and we know nothing about it,” she stated in a recent account from Bloomberg. “It could be three raccoons in a trench coat.”
The carbon emissions attributed to AI can vary widely depending on how the electricity is generated, coal or natural gas, or solar power or wind farms. Research has shown that training GPT-3 took as much electricity as 120 US homes would consume in a year. That amount of generation would produce 502 tons of carbon emissions, as much as 110 US cars exhaust in a year. And that may constitute 40 percent of the power consumed by the actual use of the model. Also, the models are getting bigger; GPT-3 uses 175 billion parameters; the predecessor model used 1.5 billion, according to the Bloomberg account.
In addition, the models need to be continually trained, in order to keep pace with reality. For example,“If you don’t retrain your model, you’d have a model that didn’t know about Covid-19,” stated Emma Strubell, a professor at Carnegie Mellon University, who was early to study the energy consumption of AI.
CodeCarbon Tools Used by Hugging Face Team
The Hugging Face team emphasized an economic use of energy overall, and they took into account in addition to the energy used to train the model, the energy needed to manufacture the supercomputer hardware on which it runs. The team used a software tool called CodeCarbon to do so; the tool tracked the carbon dioxide emissions BLOOM was producing in real time over 18 days, according to a recent account in MIT Technology Review.
The team estimated that BLOOM’s training led to 25 metric tons of carbon dioxide emissions,, and they found the figure doubled when they took into account the emissions produced by the manufacture of the needed computer equipment. The 50 metric tons of carbon dioxide emissions is the equivalent of about 60 flights between London and New York, the authors stated, but it is far less than emission associated with other LLMs of the same size because BLOOM was trained on a French supercomputer mostly powered by nuclear energy, which produces no carbon dioxide emissions.
“Our goal was to go above and beyond just the carbon dioxide emissions of the electricity consumed during training and to account for a larger part of the life cycle in order to help the AI community get a better idea of their impact on the environment and how we could begin to reduce it,” stated Luccioni in the MIT account.
Luccioni’s paper is attracting attention, since it is setting a new standard for defining the energy consumption of AI model development and use. The paper “represents the most thorough, honest, and knowledgeable analysis of the carbon footprint of a large ML model to date as far as I am aware, going into much more detail … than any other paper [or] report that I know of,” stated Emma Strubell, an assistant professor in the school of computer science at Carnegie Mellon University, Strubell was not involved in the Hugging Face study, but in 2019 she wrote an important paper on AI’s impact on the climate.
AI Can Be Employed to Help Implement Sustainable Practices
Gartner analysts predict that by 2025, AI could consume more energy than the human workforce, without sustainable practices taking hold before then, according to a recent account in the Harvard Business Review.
Fortunately, the AI can be employed to help save itself by applying some brakes to its energy consumption. “It is the application of these technologies that will identify sustainable business opportunities and drive enterprise sustainability efforts,” stated the author of the HBR account, Chris Howard, chief of research at Gartner.
“Sustainable AI practices have emerged, such as the use of specialized hardware to reduce energy consumption, energy efficient coding, transfer learning, small data techniques, federated learning and more,” Howard stated.
A recent Gartner survey found that 87 percent of business leaders expect to increase investments in sustainability over the next two years, which they see providing: protection from disruption, short- and long-term value, and opportunities to optimize and reduce costs. “Sustainability investment offers a “two for one” by supporting responsible consumption while simultaneously benefiting the business,” the author stated.
As the AI community grapples with the environmental impact of AI energy consumption, some conferences are now requiring submitters to include information on CO2 emissions, according to a recent account in IEEE Spectrum. To produce the data, researchers are using new tools and new methods to help measure emissions.
Allen Institute for AI Studied Variations in AI Energy Consumption
Two new methods are to record the energy usage of server chips powering AI as a series of measurements, and aligning this data with local emissions per kilowatt-hour (kWh) of energy used, a number that continually updates. “Previous work doesn’t capture a lot of the nuance there,” stated Jesse Dodge, a research scientist at the Allen Institute for AI, and the lead author of a new paper on the subject, presented last June at the ACM Conference on Fairness, Accountability and Transparency (FAccT), , Measuring the Carbon Intensity of AI in the Cloud Instances.
The Allen team focused on GPU usage, since they found GPUs used 74 percent of a server’s energy. Memory used a minority of the energy and supported many workloads simultaneously.
The team trained 11 machine-learning models of different sizes that were processing language or images. Training time ranged from one hour on one GPU to eight days on 256
GPUs. Energy use was recorded for every second, and carbon emissions per kWh of energy were obtained for 16 geographical regions through 2020, measured at five-minute granularity. The approach allowed the researchers to “compare emissions from running different models in different regions at different times,” according to the IEEE account.
The team found the energy required to train the smallest models emitted about the same amount of carbon as charging a phone. They trained the largest model of six billion parameters only to 13 percent of completion, and found the energy required emitted close to the amount of carbon generated by powering a home for a year in the US. OpenAI’s GPT-3 contains more than 100 billion parameters.
The biggest differences in emissions were by region. Grams of CO2 per kWh ranged from 200 to 755. The team used tools to help reduce CO2 emissions, Flexible Start and Pause and Resume. Delaying training for up to 24 hours, could save from 10 to 80 percent of the energy required for the smaller models. Pause and Resume could be used to pause training at times of high emissions; that was found to benefit larger models by up to 30 percent in half the regions.
“Emissions per kWh fluctuate over time in part because, lacking sufficient energy storage, grids must sometimes rely on dirty power sources when intermittent clean sources such as wind and solar can’t meet demand.” the article author stated.
Being able to predict emission per kWh would be helpful, according to Dodge. A researcher at the Université Paris-Saclay, a public research university based in Paris, had another idea for reducing emissions. “The first good practice is just to think before running an experiment,” stated Anne-Laure Ligozat, a coauthor of the university’s study on the energy consumption of AI development. “Be sure that you really need machine learning for your problem.”
Read the source articles and information from Bloomberg, MIT Technology Review. Harvard Business Review and IEEE Spectrum.
(Write to the editor here.)