Getting Data Centers Built Out for AI is a New Services Niche
Service suppliers are packaging AI and machine learning to offer out-of-the-box solutions for transforming data centers to better support AI processing, especially for small and medium enterprises.
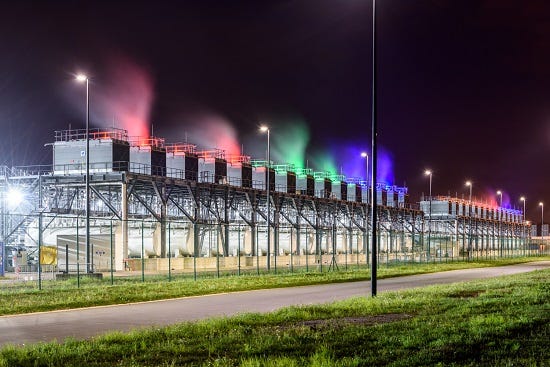
By John P. Desmond, Editor, AI in Business
Data centers are going through changes to gear up for running AI systems with different hardware and software requirements than their existing systems that may have been running for years.
For small and medium-sized enterprises, setting up a data center to support AI is a work in progress; many firms turn to service providers to package up the needed capabilities.
Still, even pre-packaged AI and machine learning solutions that are ready to go out of the box, require integration to be useful beyond a point solution. And while do-it-yourself AI deployments are doable, they require investments to collect the needed data and expertise to make them usable.
“Giant industry players have been doing it for several years already, but most data center companies are just beginning to set up their data gathering and MLOps pipelines,” stated Maciej Mazur, Product Manager for AI/ML, Canonical, in a recent account in Data Centre Dynamics.
AI is being employed by the data center itself to enhance operations. Google, for one, is providing examples of what can be accomplished by detailing how it is using DeepMind AI to assist in data center cooling. Google was able to improve power usage effectiveness by 15 percent through automatic management of fans, cooling systems and windows, according to the report.
Cooling and predictive maintenance are the most often-cited use cases for AI in the data center, according to the account, while power and workload management, and security use cases have yet to see significant traction.
“We have seen exciting innovations, particularly from organizations like Google, which is showing the huge potential AI can provide,” stated Dave Sterlace, Head of Technology, Global Data Center Solutions at ABB, the multinational based in Zurich. “The potential is there and is being demonstrated, but it’s not hugely widespread yet.”
Conversations with many customers are still centered on the early phases of defining machine learning and AIs, and the potential benefits it could bring, rather than deployment, Sterlace added.
Some See Packaged AI Solutions As a Patchwork
And AI services suppliers are still trying to figure out how to package their offerings in ways that make sense for customers, some of whom are concerned about being tied to a single vendor.
“The suppliers are providing solutions, but most are providing a solution that is specific to particular products, often from this same vendor, causing vendor lock in,” stated David Cheriton, Chief Data Center Scientist, Juniper Networks, in the Data Centre account.
The hardware and software requirements of AI are leading to solutions that could be called fragmented. “Data center operations and management solutions are still very piecemeal due to the typically heterogeneous equipment stack,” stated Michael Cantor, CIO at Park Place Technologies, a firm offering IT infrastructure support. “Different vendors are at different levels of capability, and I would say that few are embedding true AI/ML into their operations stack.”
Many companies look to retrofit their existing data centers to support AI processing, which requires the installation of many sensors. Scaleway, a company based in Paris, offers a service to retrofit data centers for AI.
“Companies considering developing their own AI/ML for data center management will need sensors in all parts of the data center to monitor temperature, humidity and electricity drawn by rack, row, cage and room,” stated Yann Lechelle, CEO of Scaleway. “In order to monitor mechanical electrical equipment, a proper information system must be put in place to log the data in an industrial way. Only then can proper data processing occur. In our latest data center, we have 2,500 sensors per room for 11 rooms.”
That’s a lot of sensors. Other hardware needed to support AI, according to a recent account in TechTarget, includes: Graphic processing units (GPUs), with their massively parallel architecture that accelerates the processing of AI models; field-programmable gate arrays (FPGAs) and application-specific integrated circuits (ASICs) are used to accelerate AI hardware processing; and more storage for the AI models and associated data.
"Most organizations that are building out training and inferencing infrastructure often quickly have a massive requirement for additional storage," stated Jack Vernon, analyst with IDC market researchers.
SambaNova Systems Has Raised $1B to Help Build the AI-Enabled Enterprise
Lots of money is being directed towards companies that can support AI operations in the data center.
SambaNova Systems of Palo Alto, Calif., for example, has raised about $1 billion in venture capital to deliver on its vision of supporting the AI-enabled enterprise. Founded in 2017, the company offers a custom-built stack of technology that include the software, computer system and processor, selling it as a service.
Cofounder and CEO Rodrigo Liang, in a recent interview in IEEE Spectrum, described the origins of the company and how it approaches its work. Discussing the impact of AI on IT and business computing, he stated, “This is the biggest transition since the internet, and most of the work done on AI is done on legacy platforms that have been around for 25 to 30 years.” And these platforms are geared towards the flow of instructions and not the flow of data, he stated.
His idea is to “flip the paradigm on its head” and design for getting the needed data where it needs to be. So instead of operators such as add, subtract, multiply, divide, load and stores, you have operators that help with the flow of data: map, reduce and filter, for example. ”These are things that are much more data-focused than instruction-focused,” Liang stated.
The company has brought the notion of hardware-software co-development to AI systems. “The first step is to take the software, break it down and see natively what you want it to do. Then you build the hardware,” he stated. The approach enables the iteration of each generation to make improvements.
The company maintains strong ties to academia, such as with scientists at Stanford, Cornell and Purdue. Its approach is difficult for competitors to emulate.
“You start with people that can produce a high-performance piece of silicon to do this type of computing, that requires a certain skill set,” Liang stated. “But then to have the skill set to build a software stack and then have the skill set to create models on behalf of our customers and then have the skill set to deploy on a customer's behalf, those are all things that are really hard to do; it's a lot of work.”
Read the source articles and information in Data Centre Dynamics, in TechTarget and in IEEE Spectrum.