How AI Helped to Produce the First Black Hole Image
EHT researchers worked with data for five years, using AI to help filter out galactic noise, to produce the first actual measurement of a black hole that can be compared with predictions.
By John P. Desmond, Editor, AI in Business
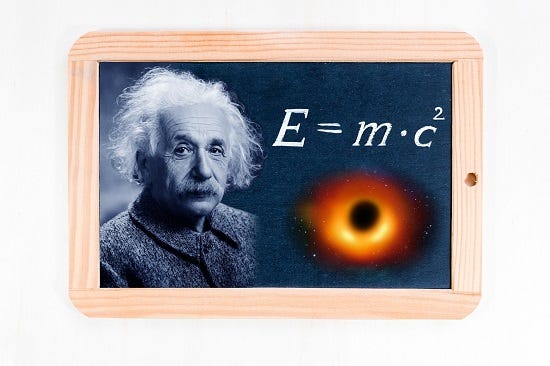
AI techniques were pivotal to the ability of an international team of more than 300 scientists from 80 institutions to create the first-ever image of the supermassive black hole at the center of the Milky Way galaxy.
The image, called Sagittarius A* (Sgr A*), was produced by the Event Horizon Telescope (EHT) collaboration, using observations from a worldwide network of radio telescopes.
Scientists had previously seen stars orbiting around something invisible, compact and massive at the center of the Milky Way, strongly suggesting that the object is a black hole. The newly released image provided the first direct visual evidence that is the case.
“Being able to see the shadow of the black hole, the gas flowing around it and the blackness at its heart, is extraordinary,” stated Shami Chatterjee, principal research scientist at the Cornell Center for Astrophysics and Planetary Science and a member of the EHT collaboration, in a recent account in the Cornell Chronicle . “We can do a lot of physics with this data – for the first time we have an actual measurement, and we can compare it with predictions from general relativity,” he stated.
The black hole is about 27,000 light-years away from Earth. It appears from Earth to have about the same size in the sky as a doughnut on the moon. To capture the image, the team created the powerful EHT, which linked together eight existing radio observatories across the planet to form a single “Earth-sized” virtual telescope. The EHT observed Sgr A* on multiple nights, collecting data for many hours in a row, similar to using a long exposure time on a camera.
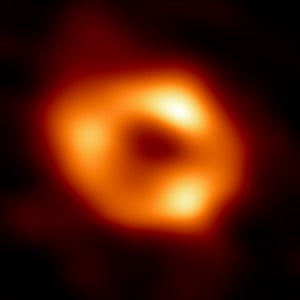
The team worked for five years, using supercomputers to combine and analyze their data, while at the same time compiling a library of simulated black holes to compare with the observations. The group relied heavily on machine learning and AI, according to Chatterjee, because of the noisiness of the data.
“With so many telescopes, there’s a lot of interference – from cell phones, from satellites passing overhead, from the telescope slewing back and forth – and all of that has to be filtered out while we look for an astrophysical signal of interest,” stated James Cordes, professor of astronomy at Cornell and a member of the EHT collaboration. “It’s like looking for a needle in a haystack, so it’s a place where machine learning can and is playing a significant role. The machine learning identifies interesting signals and we humans can inspect them. It reduces the problem of the massive onslaught of interference into manageable proportions.”
Light cannot escape a black hole, but hot plasma swirling around it emits short radio waves that radio telescopes can pick up. In the image, the gas silhouettes the black hole itself. “For decades, astronomers have wondered what lies at the heart of our galaxy, pulling stars into tight orbits through its immense gravity," stated Michael Johnson, an astrophysicist at the Center for Astrophysics, in a press release quoted in an account from Axios.
Einstein's theory of general relativity predicts the existence of black holes. Scientists theorize that in some instances, general relativity will not hold for black holes, which could require a new type of physics to describe.
Matthew Strassler, a theoretical physicist at Harvard (not involved in EHT) conducts experiments to detect gravitational wave signals from colliding black holes and neutron stars.
"Anytime you open up a new window into some type of complex physical phenomena, you can not predict what surprises might lie in store," he stated. Opportunities to ask new questions may present themselves as the black hole imaging measurements become sharper, he added.
The EHT researchers collected their data on five nights in April 2017. They revealed their first black hole image, of M87, in 2019, showing the first direct evidence of the spherical shape that shrouds a black hole’s exterior. The researchers collected nearly 4 petabytes (4,000 terabytes) of data, which was too much to be sent over the internet and had to be carried by airplane on hard disks, according to a recent account in nature.
To train the AI algorithm at the heart of EHT, the team fed it with lots of galactic and other images to make it learn how things look in the universe, stated Deepak Singh, AI scientist and product developer and manager, writing in Medium in the fall of 2020, after the Nobel prize in physics was awarded to three black hole researchers.
This data combined with the data collected by the eight telescopes helped to build the first-ever image of a black hole. The team at MIT along with researcher Katie Bouman, an assistant professor in the Computing and Mathematical Sciences Department at the California Institute of Technology, took a different approach to train the algorithm. “They trained the algorithm on three different sets of data,” Singh stated, adding that, “I am pretty optimistic that we will soon be able to take the picture of this black hole and determine its size, composition, and physics around it.”
And that came to pass two years later.
Commenting on the discovery in a release from Nasa’s Ames Research Center, Bouman stated, “We have developed [a method] to photograph a black hole using the Event Horizon Telescope, a network of telescopes scattered across the globe. Imaging a black hole's structure with this computational telescope requires us to reconstruct images from sparse measurements, heavily corrupted by atmospheric error.”
At a press conference in Washington, DC announcing the recent discovery, according to the account in nature, Bouman stated, “We’ve been working on this for so long, [that] every once in a while you have to pinch yourself and remember that this is the black hole at the center of our Universe. What’s more cool than seeing the black hole at the center of the Milky Way?”
Read the source articles and information in the Cornell Chronicle, from Axios, in Medium, from Nasa’s Ames Research Center and in nature.
(Write to the editor here.)