HPC With AI Shows Promise for Enabling Lights Out Data Centers
HPC is seen as a needed platform for automated data centers; predictions vary on numbers of on-premise data centers to remain; data center ops staff are being advised to diversify their skill sets.
By John P. Desmond, Editor, AI in Business
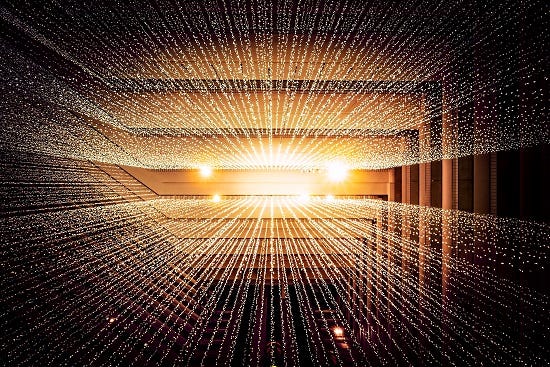
High-Performance Computing (HPC) originally referred to supercomputers but today refers to computing clusters and grids, accessible by a collapsed network backbone, in which troubleshooting and upgrades can be applied to a single router.
HPC working with AI shows promise for a range of use cases, including, potentially, unmanned data centers.
The data center as a use case for HPC has appeal, since it can be applied to so many aspects of a successful lights-out data center operation, and shows promise as a greener alternative.
“More specifically, the IoT and artificial intelligence (AI), backed by the power of hyperscale computing, have become important tools for enabling lights out (unmanned) data center operation,” stated Sameh Yamany, Ph.D., chief technology officer for Viavi Solutions, writing recently in Data Center Dynamics. Viavi supplies a range of network automation services.
Hyperscale data centers in the view of IDC analysts need a 10,000 square foot minimum footprint, in an era where many data centers exceed one million square feet. This vast unmanned data center needs automated temperature sensing and response, fire protection, remote automation, power monitoring and security. “Each contributing element also improves efficiency,” Yamany stated. “This includes optimized power, cooling, and security based on real-time adjustment and the removal of geographic constraints.”
The transition of HPC systems to graphic processing units (GPUs) for the bulk of their processing, has helped to meet the demands of AI systems for huge volumes of data for storage, processing and transfer, suggests a recent account in Technative.
“HPC’s GPU parallel computing has been a real game changer for AI,” with parallel computing able to process data in a shorter amount of time, stated Vibin Vijay, AI and ML product specialist at OCF, author of the account. OCF, based in Sheffield, England, provides HPC, storage, cloud and AI services
In an example from image analysis, one GPU would take 72 hours to process an imaging deep learning model. An HPC cluster with 64 GPUs could do the work in 20 minutes, Vijay stated.
HPC clusters can speed the development of AI projects. “Training an AI model takes far more time than testing one. The importance of coupling AI with HPC is that it significantly speeds up the ‘training stage’ and boosts the accuracy and reliability of AI models, whilst keeping the training time to a minimum,” Vijay stated.
For simulations run on HPC platforms, AI models can be used to predict outcomes without having to run a full, resource-intensive effort. Input variables and design points of interest can be narrowed down to a candidate list quickly, at a much lower cost, Vijay suggests.
HPC architecture is needed to support the demands of AI systems, suggests a recent account on the blog of Liqid, suppliers of what the company calls a “composable infrastructure hardware and software platform.”
“Traditional data center infrastructures based on fixed, inflexible server architectures, simply cannot perform at the level these applications require,” stated the authors (who were not identified specifically),” with performance and capacity essentially fixed at the point of sale. But with workloads that require real-time responsiveness from hardware resources, static architectures hit a wall.”
Research continues into the development of HPC systems. For example, the Texas Advanced Computing Center (TACC), located at the University of Austin, is in the process of designing and deploying its Horizon HPC system, which is funded entirely by the National Science Foundation (NSF) to support its compute efforts across domains.
“Built into the core of the Horizon project is a mandate to come up with a more sustainable compute model than the competitive refresh cycles that sees one system abandoned for fully new hardware every few years in order to reap the performance rewards,” state the Liqid blog authors. One of the project requirements is that the system perform 10x faster than TACC’s current HPC production environment.
To begin, the team solicited the IT community for the applications they believe will be core to scientific computing in the next several years. The team is designing systems that can support those applications.
A range of hardware and software resources are under consideration for their ability to provide a platform that can deliver on the performance requirements while also delivering a more sustainable, flexible growth model. It needs to “incorporate vendor-neutral technologies as they emerge without having to refresh the system as a whole in order to reap evolving performance rewards,” the blog authors suggest. AI runs on the platform, and is also used to improve efficiencies at the “bare-metal level” by observing data over time and making adjustments to optimize operations.
The move to lights-out data centers and incorporation of AI tools and techniques to manage data centers, as well as run AI systems, also presents challenges and opportunities for data center operations staff.
"Data center professionals can prepare for the AI computing transition by increasing their knowledge of AI and machine learning as applied to application use cases to enable the organization to generate new services and products to gain competitive advantage in the market," stated Rodrigo Liang, co-founder and CEO of SambaNova, in a 2021 account from ZDNet, "Unlike other technology trends that existed to drive down costs, AI represents a huge opportunity to generate value -- and it is being driven by all the data being generated by every computer and sensor on the planet." SambaNova provides services for the “AI-enabled enterprise.”
A survey of 500 IT executives conducted by INAP in 2020 found that 85 percent of professionals anticipated their data centers will be close to full automation by 2025. Research varies on the percentage of companies that will maintain on-premise data centers, but advice is consistent for data center professionals to diversify their skill sets and be flexible.
To be successful in data center roles today, data center managers and professionals "need to be critical thinkers, fast learners, and adept at maintaining situational awareness," stated John O'Connor, manager of technology infrastructure operations at Bloomberg, in the ZDNet account. “Problems occur less often, but tend to scale quickly and are more difficult to triage and remediate. They need to keep a massive amount of context in their heads and be able to leverage all the telemetry and IoT data available to them to derive the problem scope and evaluate potential solutions quickly."
With higher data center automation and the continuing move to cloud computing, data center jobs are likely to change but are unlikely to be eliminated.
Companies that have made big moves to the cloud "have typically found other roles for people working in data centers that did easier things, such as changing tapes, hard drives, or working with physical equipment," stated Steve Jones, DevOps advocate at Redgate Software. "The DBAs still have to work with the databases, though their role has changed. They don't do some of the hardware-related tasks or patching, but they do need to manage security, check on performance, and many other similar tasks."
Read the source articles and information in Data Center Dynamics, in Technative, on the blog of Liqid, from ZDNet and on the site of INAP.
(Write to the editor here.)