Industrial AI and Industrial AIoT Seen Reshaping Manufacturing in 2022
Drivers include a generational shift in the workforce, the emergence of industrial data scientists, precise industrial AI models and executive ownership to drive deployments.
By John P. Desmond, Editor, AI in Business
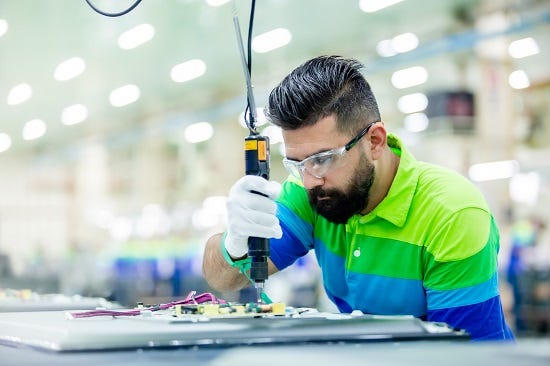
Industrial AI is seen as reshaping manufacturing for its potential to automate and reinvent some fundamental industrial processes, including product development and manufacturing, and supply chain operations.
Fueling this trend is a generational shift in the workforce, as the Great Resignation creates openings and more veteran employees hit retirement age. New hires out of college have different knowledge and experiences to contribute.
“Often, these new graduates come to the job having learned technologies and concepts in school that don’t match the reality of how the workflows and systems on the plant floor actually operate,” stated Bill Scudder, a VP and General manager at AspenTech, writing in a recent account in RT Insights on four ways industrial AI is seen as reshaping manufacturing. Headquartered in Bedford, Mass., AspenTech provides software and services for the process industries.
This new generation will prompt an acceleration in adoption of knowledge automation technologies, helping to close the skills gap between the departing workers and new ones, and preserving the domain knowledge. It will also make the manufacturing jobs more appealing to potential recruits, he suggests.
In addition, Scudder sees that industrial data scientists will emerge to facilitate the industrial AI strategy. He cited Aspen research showing that 84 percent of key industrial decision-makers see the need for an industrial AI strategy, but only 35 percent have deployed such a strategy so far. “With one foot in traditional data science and the other in unique domain expertise, industrial data scientists will serve a crucial role,” he stated.
He also suggests that AI investments will shift from generic models to more precise industrial AI models. These fit-for-purpose, precise industrial AI applications will help companies achieve profitability and sustainability goals. “This means moving away from AI models trained on large volumes of plant data that cannot cover the full range of potential operations,” he stated, adding, “Industrial data will be transformed into real business outcomes across the full asset lifecycle.”
This more specialized focus for industrial AI will require a larger set of solutions providers, pooling their expertise, he suggested, noting, “This will place a greater focus on time-to-value partnerships, helping to lower the barrier to AI adoption more than ever.”
Lastly, Scudder sees that executive ownership will accelerate industrial AI deployments. Chief Digital Officers will be appointed to overcome obstacles and shepherd digital transformation and industrial AI through the organization, helping to bridge the gap between legacy systems and new technologies.
The market for AI in manufacturing is projected to reach $16.7 billion by 2026, with growth rates of over 50 percent a year, according to Global AI in Manufacturing Market Trends, quoted in a recent account in Robotics & Automation.
The top five use cases for AI in manufacturing are seen as:
Predict design failures;
Assess quality in product manufacturing;
Engage in predictive maintenance;
Effective demand and price forecasting; and
Inventory management.
For assessing quality, “AI can identify even minor faults in machinery or product,” stated the author, Mark Allinson, editor of the publication, adding, ”Artificial Intelligence is powering manufacturing processes in radical ways.”
In another account, IoT Analytics, reports the AI adoption rate in industrial settings has increased from 19 percent to 31 percent in slightly more than two years.
“Increased AI adoption can be witnessed across the board, but is especially strong in the energy vertical and in process industries, such as oil and gas or chemicals,” stated the author, Fernando Brügge, an analyst with IoT Analytics, a market research firm based in Hamburg, Germany.
The firm’s related report, entitled “Industrial AI and AIoT Market Report 2021-2026,” forecasts the market that includes manufacturing and energy companies globally, will reach $102.2 billion by 2026, with growth rates of 35 percent annually. AIoT is defined as a subset of industrial AI that refers to AI performed on IoT-type data sources in industrial enterprises.
The authors identified four soft-related trends driving widespread adoption of AI within industrial settings:
Software tools are increasingly available for industrial AI. IoT Analytics identified 634 vendors offering AI technology for manufacturing clients; of those, 389 offered AI software;
New AI software platforms focus on industrial settings. Examples include Genix from ABB, FactoryTalk from Rockwell Automation; and Autonomous Production Advisor from Schneider Electric.
Hyperscalers are extending their offerings to the shop floor. These include AWS, Microsoft and Google, with offerings such as SageMaker from Amazon, which offers pre-built and customizable solutions for common industrial use cases, such as predictive maintenance.
Use case-specific software solutions are driving increased availability. These solutions include, again, predictive maintenance tools, which numbered 73 in early 2021 due to an increase in the variety of data sources and the use of pre-trained models.
In addition, industrial AI is being supported by improved hardware, the author stated. This includes AI chips, such as NVIDIA’s latest A30 and A10 chips, and Google’s fourth generation of tensor processing units (TPUs), which are application-specific integrated circuits (ASICs), used to achieve greater efficiency and speed for specific AI workloads.
“The use of dedicated AI hardware reduces model calculation time from days to minutes and has proved to be a game changer in many instances,” stated author Brügge.
Hindalco Industries Employs Rockwell Automation Solution to Digitize
A case history from Rockwell Automation details a digital transformation experience at the world’s largest aluminum rolling company, Hindalco Industries of Mumbai, India.
The company was seeking real-time visibility into data siloed within operational technology systems, which were labor-intensive and complex to work with. It could take days or weeks at some facilities to extract the needed data, hampering analytics initiatives meant to optimize processes and improve production efficiency.
Chief Digital Officer Jagdish Ramaswamy decided to form a core team of experts to drive a digital transformation at Hindalco. The goal was to:
Create smarter plants by providing real-time visibility into operations and key performance indicators, access data without barriers, and use AI and machine learning to improve asset reliability and product quality;
Improve supply chain efficiency by optimizing procurement costs using ML and price forecasting models;
Develop a digital-savvy workforce and deploy cutting-edge technologies.
The team recruited more than 75 early-career professionals from across all functions that they called Digital Ambassadors. This team helped to increase adoption of a digital culture, by running sessions on how to use digital transformation to solve problems, by justifying the needed investments and by communicating the return on investment.
“We started a program called DISHA: Digital Shiksha. Shiksha is a Hindi word for education,” stated Ramaswamy. “Our partners helped teach our people how technology works. Over the last two years, we have done more than 15 digital immersion sessions for more than 800 people to spread awareness of the value of digital technologies for the business.”
Among examples of business benefits of the transformation, Ramaswamy cited:
Coil quality is known hours after a sample is taken from the end of a coil;
An ML model is suggesting optimum levels to operate the process, which is improving on-time delivery and reducing non-conformance percentages;
Improved smelter processes that reduce production costs;
A digital twin is used to identify critical factors that contribute to efficiency and production improvements,
Read the source articles and information in RT Insights, in Robotics & Automation, in IoT Analytics and in a case history from Rockwell Automation.
(Write to the editor here.)