Power of Large Language Models Being Tried as Warnings Issued
Software supplies are working to tap the power of LMMs to seize new revenue opportunities, as one warning is issued that the impact of higher automation on the internet is unknown.
By John P. Desmond, Editor, AI in Business
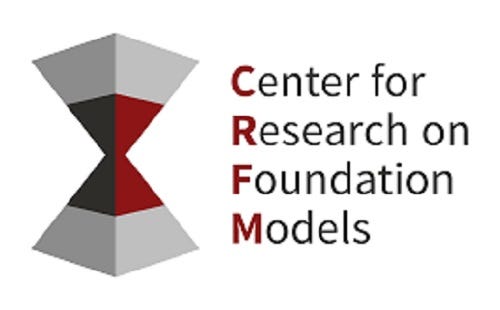
Software and service suppliers are running with the ball in efforts to tap the power of AI large language models (LMMs) to create new revenue opportunities, as even larger LMMs loom on the horizon and warnings are issued on the potential risks that LMMs pose.
Natural language processing advances are marking the progress of AI, such as with OpenAI’s GPT-3 LMM, which is said to be capable of producing text as if from a human.
This suggests that “auto-generated articles that are indistinguishable from human writing, improved real-time language translation and meta-learning capabilities are just a few ideas of what might come next,” stated Michael Krause, senior manager of AI Solutions at Beyond Limits, a company offering what it calls “actionable AI,” combining machine learning and knowledge-based reasoning, in a recent account from datanami.
LMMs will need huge volumes of data to train themselves, which is boosting the market for synthetic data as a promising new source.
“Early implementations for generative AI technology lets companies do things like leverage identity marketing content with a higher success rate, and leverage highly nuanced NLP capabilities to diagnose health cases through text and image data,” stated Wilson Pang, CTO of Appen, a company focused on improving data used to develop machine learning applications. “We may see more use cases emerge over the next year as experimentation and adoption picks up,” Pang stated.
LMMs are trained on enormous sets of publicly-available generic texts that are crawled and clawed from all over the internet. One company sees an opportunity to produce more focused data sets. “We see a gap between what models trained on generic data can do versus what a model trained on a company’s domain-specific data can do,” stated Natalia Vassilieva, director of product for machine learning at AI hardware maker Cerebras, which offers an AI accelerator.
The company is working on closing that gap. “We expect that an ability to continuously pre-train, or fine-tune, these gigantic generic language models with proprietary domain-specific data will be of high interest and, once trained and deployed, will deliver better insights to domain scientists,” she stated in the datanami account. “In 2022 we will need to figure out how to do that efficiently, and also how to reduce the cost of running predictions with these humongous models once they are trained and tuned. Pruning and distilling the models might be a way to do so, as well as relying on a special-purpose hardware.”
LMMs are capable of producing synthetic characters, which are expected to advance beyond what we see in chatbots today.
“We will see the growth of a new hybrid workforce in which human employees share their workload with digital employees,” stated Natalaie Monbiot, head of strategy for Hour One, which provides synthetic characters based on real people. These characters can potentially “offload repetitive or routine tasks to machines that can perform them just as well, and in some cases better,” Monbiot stated.
Or, employees could create digital avatars with superhuman skills, such as the ability to speak any language. “This will serve to break down geographical and cultural barriers and enable a whole new era of frictionless communications,” Monbiot suggests.
More powerful listening is also on tap. “Voice is the most natural form of communication. However, machines have historically been locked out of listening and analyzing conversations,” stated Scott Stephenson in the datanami account. Stephenson is the CEO and cofounder of Deepgram, providing automated speech recognition software.
He sees an opportunity in having the language models better understand how words are said, as well as what is being said, to help understand what customers want and to empathize with them. “Reducing bias in speech infrastructure will also be a top priority for vendors so that their customers can more accurately understand the voices of various backgrounds, genders, and languages of their users,” Stephenson stated.
Coming GPT-4 To Focus More on Coding
The GPT-3 model with its 175 billion parameters is about to be superseded by the GPT-4 model, which is expected to be more focused and not necessarily larger, according to Sam Altman, the CEO of OpenAI, from an account in Analytics India Magazine.
Conventional wisdom suggests that the more parameters a model has, the more complex tasks it can achieve, but some researchers are suggesting this may not be the case. A group of researchers at Google, for instance, recently published a study that showed a model much smaller than GPT-3, a fine-tuned language net (FLAN), achieved better results than GPT-3 on a number of challenging benchmarks.
GPT-4, with no announced release date, will focus more on coding, Alrtman stated, with a capability called ‘Codex,’ which today is the basis for GitHub CoPilot. Codex understands more than a dozen languages and can interpret simple commands in natural language and execute them, which allows a natural language interface to existing applications.
LMMs To Have an Unknown Effect on the Internet
A warning about the potential negative effect of large language models such as GPT-3 and the forthcoming GPT-4 on the internet was sounded recently by Michael Kevin Spencer, writer and technology critic, in a recent account in Data Science Central.
“The NLP explosion of GPT-3 like technologies will be able to automate content at scale, and currently we have no idea how this could impact the internet,” Spencer stated.
“The incentives that current algorithms provide already have led to a perverse internet and dopamine loop induced feed scrolling zombies and an entire generation or two that have grown up with the internet and mobile phones, instead of a normal social life,” Spencer stated. “We’ve engineered a generation built to consume on the internet and the next generation of AI and GPT-3 like technologies are only going to make it worse.”
The quality of content is getting worse, and now we enter an area where more of it will be machine-generated and not about real humans, Spencer suggested.
A group of researchers at Stanford University concerned that LMMs could be exhibiting widespread biases and other flaws have formed the Center for Research on Foundation Models (CRFM), an initiative of Stanford’s Institute for Human-Centered Artificial Intelligence (HAI).
Their concern is that GPT-3 and similar efforts are foundation models that create a “single point of failure” with any defects or biases carried downstream and “blindly inherited.”
Fei-Fei Li, former director of Stanford’s AI lab and now codirector of HAI, sees that the profit motive is encouraging companies to “punch the gas” in the absence of AI ethics and regulation, instead of braking for reflection and study.
Read the source articles and information from datanami, Analytics India Magazine and in Data Science Central.