Privacy Engineering Making Headway with Help From AI Platforms
Privacy engineering is an emerging field that employs AI tools and techniques to design privacy into AI systems from the ground up. Standards and best practices are being compiled.
By John P. Desmond, Editor, AI in Business
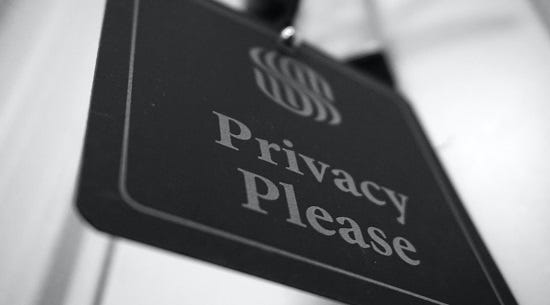
Security leaders making strategic privacy decisions need to balance the tradeoff between leveraging personal data and maintaining privacy, in what is being described in more circles as a privacy engineering challenge.
The fast pace of processing power growth and the emergence of innovative technology incorporating AI is causing a change in user expectations and demands. “The fast-paced change is causing a seismic shift in privacy expectations of users and regulators,” stated Katharina Koerner, head of IAPP’s Privacy Engineering Section, in a recent account she authored in Security Magazine.
“It is a constant challenge for organizations to ensure they are evolving their privacy-by-design practices to meet expectations,” stated Koerner, who brings together engineers, data scientists, US designers and other technologies to build privacy into products and services for the non-profit IAPP, the International Association of Privacy Professionals, headquartered in Portsmouth, New Hampshire.
Data protection regulations, including the EU’s General Data Protection Regulation, refer to “state of the art” as the guide for technical design choices. State of the art implies mature scientific knowledge and research, and a reference to international standards. “Often, state-of-the-art technology is expensive and complex to deploy,” she stated.
As a result, organizations need to acquire the right privacy engineering skills, which is likely to require an investment in the workforce. This translates to support, resources and training for security professionals and privacy engineers.
Regulators are soon expected to issue opinions on legal questions on new approaches, such as anonymization techniques spurred by the new GDPR guidelines. These techniques may be applied to international data transfers and cross-border medical research, for example, in order to comply with the new privacy guidelines.
Big tech companies, including Apple, Google, Facebook and Microsoft, are leading the adoption of privacy-enhancing techniques. These include differential privacy – “one of the strongest guarantees of privacy available,” Koerner states – a system for publicly sharing information about data by describing patterns while withholding information about individuals.
Going forward, “The key will be … responsible use of data in AI and privacy by-design in AI systems,” Koerner stated.
Privacy Engineering Field is Emerging
Privacy engineering is an emerging field of engineering, in which practitioners try to implement privacy laws and best practices in information systems. “One reason for the lack of attention to privacy issues in development is the lack of appropriate tools and best practices. There are few building blocks for privacy-friendly applications and services,” states an entry on privacy engineering in Wikipedia.
“This climate of change begs a climate of innovation,” stated Sabarinathan Sampath, Senior Vice President and COO, ZNet Technologies, author of a recent account in Wire19. “The goal of privacy engineering is to make Privacy by Design the de-facto standard for IT systems.”
Consumers want personalized content and service delivery, and at the same time, they want privacy to be protected and maintained at all costs. They expect organizations and businesses to take action to protect consumers, and they want the government to protect the data of citizens.
Consumers want transparency about how businesses are storing and using their data. “They are very concerned about how their personal information is used by advanced technologies like AI,” Sampath stated. “Many consumers don’t trust that businesses will keep their data secure. Once trust is lost, consumers take action to protect themselves and their data.” This could be by switching companies or providers, to move to ones they trust.
The challenges facing effective privacy engineering Sampath sees as including:
Inability to protect data one does not know about. Data proliferates across the cloud, managed service providers and on premise in most organizations. “The challenges lies in locating the data, understanding where it originated from, and tracking it in a dynamic environment,” he stated;
Baking data privacy into the core system design of legacy systems;
Resolving the tug-of-war between data privacy and data usability;
Absence of standards or best practices for integrating privacy into the software systems development lifecycle.
Sampath suggested these best practices can help meet the challenges:
Conduct a privacy impact assessment across the organization to understand the purpose of the collection of personal data;
Understand how and why cookies are used;
Issue a privacy policy to customers that is easy to understand;
Work to establish a trust framework across people, processes and technology.
“Privacy engineering, like the privacy profession, is a constantly evolving discipline,” Sampath stated.
Progress is being made. The National Institute of Standards and Technology (NIST), a non-regulatory agency of the US Department of Commerce, issued Version 1.0 of its Privacy Framework in January 2020.
In December, Sampath participated in a panel on Privacy Engineering at the Annual Information Security Summit, along with these panelists;
Ivana Bartoletti, Global Chief Privacy Officer, Wipro;
Nitin Dhavate, FIP, CIPP(E), CIPM, CISSP, CISM, Country Head for Data Privacy, Novartis;
Ratna Pawan, Transformation Director – Risk Advisory, EY; and
Tejasvi Addagada, Data Protection Officer, Axis Bank.
The lineup reflects the advance of privacy engineering within enterprises.
Privacy-Engineering-As-a-Service Startup Gretel Labs Raises Another $50 Million
Now the segment is attracting capital. Privacy engineering-as-a-service startup Gretel Labs last fall announced last Fall that it has raised $50 million in new funding to fuel innovation, accelerate growth and expand into new use cases linked to data privacy, according to an account in SiliconAngle.
Founded in 2020, Gretel offers a platform for testing anonymized versions of a data set automatically. The platform uses AI and machine learning techniques to provide synthetic data, saving time for engineers. With its new funding, Gretel plans to continue to advance the AI capabilities of its platform to support use cases in the life sciences, financial, gaming and technology industries.
“The drive behind the last two decades of investment in cloud-native and developer tooling has been to power high-velocity development in ML/AI, IoT and all applications which require access to enormous volumes of data that is bound by ethics, privacy regulations and public trust,” stated Ali Golshan, co-founder and chief executive officer of Gretel.ai, in a statement. “At Gretel, we are building tools that enable privacy by design, which in turn provides fast and easy access to data that fuels innovation with privacy by building it into the fabric of applications.”
Read the source articles and information in Security Magazine, in Wire19 and in SiliconAngle.
(Write to the editor here.)