Reduce ChatGPT Hallucinations With Prompt Techniques
Attorney gets in trouble after not verifying his brief, which contained “made up” cases; OpenAI working on it; a content editor with ChatGPT experience offers suggestions
By John P. Desmond, Editor, AI in Business
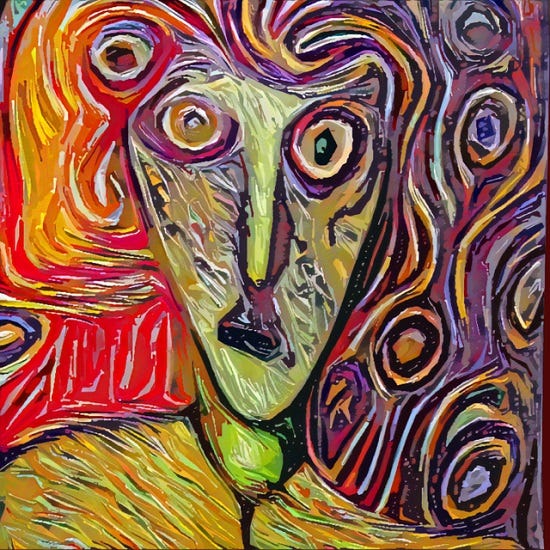
Attorney Steven Schwartz used ChatGPT to help research a case, then was cited by the court for submitting fake cases in a legal brief for a proceeding in Federal District Court. The New York Times headline called him “the ChatGPT lawyer.”
“I did not comprehend that ChatGPT could fabricate cases,” he said to the judge, according to an account in The New York Times.
Hallucination in AI refers to a confident response not justified by the training data used in the above case for ChatGPT. Some researchers are not comfortable with the term, believing it anthropomorphizes AI in an unreasonable way, according to an account on Wikipedia.
Writing on his own website, business and technology writer Bernard Marr cited four reasons that hallucinations are a problem for AI: erosion of trust, ethical concerns, impact on decision-making and legal implications, as Steven Schwartz can attest.
Regarding decision-making, Barr stated, “AI systems are increasingly used to inform critical decisions in fields such as finance, healthcare, and law. Hallucinations can lead to poor choices with serious consequences.”
OpenAI is working on ways to combat hallucinations by ChatGPT’s models, which “exhibit a tendency to invent facts in moments of uncertainty,” an OpenAI researcher stated in a paper, according to an account from CNBC. “Detecting and mitigating a model’s logical mistakes, or hallucinations, is a critical step.” stated Karl Cobbe, an OpenAI researcher. “The motivation behind this research is to address hallucinations in order to make models more capable at solving challenging reasoning problems,” he stated. OpenAI plans to submit the research paper on its approach to mitigating hallucinations for peer review.
OpenAI Trying a Human Feedback Loop to Combat Hallucinations
OpenAI is using a technique called reinforcement learning with human feedback (RLHF) to try to improve the accuracy of its large language models, according to a recent account in IEEE Spectrum. RLHF was developed by OpenAI and Google’s DeepMind team in 2017 as a way to improve reinforcement learning. OpenAI is employing an iterative process, which periodically involves a human, to adjust and hopefully improve the behavior of its models.
“I’m quite hopeful that by simply improving this subsequent reinforcement learning from the human feedback step, we can teach it to not hallucinate,” stated Ilya Sutskever, OpenAI’s chief scientist and a creator of ChatGPT, to IEEE Spectrum
.However, AI scientist Yann LeCun, whose research on neural networks is relied on to a degree in large language models, is skeptical. “Large language models have no idea of the underlying reality that language describes,” he stated to IEEE Spectrum. “Those systems generate text that sounds fine, grammatically, semantically, but they don’t really have some sort of objective other than just satisfying statistical consistency with the prompt.”
He added, “Language is built on top of a massive amount of background knowledge that we all have in common, that we call common sense.” Computers may need to learn by observation to gain similar knowledge, he suggested.
“There is a limit to how smart they can be and how accurate they can be because they have no experience of the real world, which is really the underlying reality of language,” stated LeCun. “Most of what we learn has nothing to do with language.”
Sutskever of OpenAI pushed back a bit on the idea that the test falls short of expressing the world. “Our pretrained models already know everything they need to know about the underlying reality,” he stated to IEEE Spectrum.
Some see an opportunity in the limited ability of LLMs to be precise. Diffblue is a company that uses reinforcement learning to automatically generate unit tests for Java code, saving time for developers. CEO Mathew Lodge stated the company’s systems “can be vastly more accurate than LLMs to the point that some can work with minimal human review.”
He sees LLMs as being well-suited for freewheeling creative interaction, but that the models are highly unpredictable and making them larger does not fix the problem. “LLMs are best used when the errors and hallucinations are not high impact,” he stated to IEEE Spectrum.
Tips for Tailoring Prompts to Cut Hallucinations
Preventing ChatGPT and other LLMs from hallucinating is now a branch of AI development. Elena Alston, content specialist of London-based Zapier, offering a product for integrating web applications and automating workflows, wrote recently on the blog of Zapier about top ways to prevent hallucinations. Most have to do with “prompt engineering.” They include:
Limit the possible outcomes: When giving the LLM instructions, limit the outcomes by specifying the type of response you want, as in, answer yes or no, or choose from a specific list of options;
Pack in relevant data and sources unique to your company: As the jury is prepared during a trial to decide the outcome, ground prompts with relevant data and information, to allow the AI to generate a more sophisticated response and avoid hallucination.
For calculations, create a data template. The model is better behaved and less prone to “bad math” if provided with example data for calculations. For example, instead of writing a prompt in text format, generate a data table that serves as a reference for the model to follow.
“This can reduce the likelihood of hallucinations because it gives the AI a clear and specific way to perform calculations in a format that's more digestible for it,” Alston wrote, adding, “There's less ambiguity, and less cause for it to lose its freaking mind.”
Give the AI a specific role and tell it not to lie. For example, tell the model it is one of the best mathematicians or historians in the world, followed by your question. “If you ask GPT-3.5 a question without shaping its role for the task, it will likely just hallucinate a response,” Alston wrote, adding, “You can also tell the AI that if it doesn't know the answer, then it should say so instead of trying to invent something. That also works quite well.”
Tell the model what you want, and what you don’t want. The phrases “exclude all fictional mentions” and “return only real results,” can work. “Of course, I'm predicting by now that the AI will get sloppy with its version of events, so by preemptively asking it to exclude certain results, I get closer to the truth,” Alston stated.
Verify, verify, verify. “To put it simply, AI is a bit overzealous with its storytelling,” Alston stated, acknowledging that OpenAI and other LLM suppliers are aware of the problems with hallucinations and are working on developing new models that require more human feedback. Still, “AI is still very likely to fall into a comedy of errors,” she stated.
So whether using a generative AI model to write code, problem solve or carry out research, “Refining your prompts .., can help it do its job better, but you still need to verify each and every one of its outputs.”
After his experience with ChatGPT, attorney Steven Schwartz can now attest to the value of that approach.
Read the source articles and information from The New York Times, on the site of writer Bernard Marr, from CNBC, in IEEE Spectrum and on the blog of Zapier.
(Write to the editor here; tell him what you want to read about in AI in Business.)