Scaling AI a Top Trend of 2022 as AI Value Proves Out
Experience with a “pilot-palooza” of failed AI projects is teaching valuable lessons, resulting in a move to best practices and adoption of supporting tools such as an MLOps platform.
By John P. Desmond, Editor, AI in Business
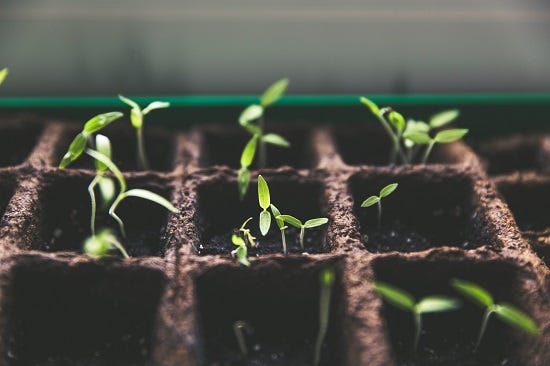
Scaling AI is a top trend of how AI is being used in business in 2022, with organizations seeking to roll out AI projects that demonstrate value more widely.
It is setting the stage for a big scaling of AI. “The human skills are present, the tools are cheaper, and it’s easier now to get access to data that might be relevant to what you’re trying to accomplish,” stated Whit Andrews, vice president and distinguished analyst at Gartner Research, in a recent account in VentureBeat.
Another observer sees that it is success with tactical or localized AI projects that is leading to a demand for scaling. “We’re at the point where a lot of enterprises are now seeing the value in AI, and they want to do it at scale,” stated Sameer Maskey, founder and CEO at Fusemachines and an adjunct associate professor at Columbia University. Fusemachines offers AI services and education.
John Deere, the agriculture company, has come to epitomize how to do AI right. The AI technologists at Deere concluded from early AI experiences that it was all about the data. “Everybody’s trying to figure out how to go to the next level,” stated Julian Sanchez, director of emerging technology at Deere. “I know that the only way John Deere got there was through a rigorous and extensive process of data collection and data labeling.” The company worked hard on techniques to get the right data collected and put to use “in a way that is not too onerous,” he stated.
Another observer sees a conflict between adding more AI pilot project use cases, and scaling the successful ones. Di Mayze, global head of data and AI at agency holding company WPP, a British multinational advertising and PR agency, as “pilot-palooza.” She stated, “They’re trying to find ways to link all the various trials to enable a scaled AI capability, but companies are realizing they need to get their data in order before they can worry about scaling AI.
Current trends related to scale include: use of synthetic data combined with real data; use of large-scale AI models; the growing use of MLops (machine learning operations) platforms, to bring coherence to deployment strategies; and awareness of scaling AI responsibly.
Efforts to Scale AI Leading to More Disciplined Deployment Practices
Successful projects incorporating AI are driving the move to scale AI more widely across organizations. An example project at Dow Chemical was cited in an account headlined, “How to Scale AI In Your Organization,” published recently in Harvard Business Review. The account was written by Manasi Vartak, founder and CEO of Verta, which offers an MLOps platform.
Dow Chemical used machine learning to accelerate its R&D process for polyurethane formulations by 200,000 times. That took it from taking two to three months to just 30 seconds.
These experiences are leading to a prediction from Gartner analysts that more than 75 percent of organizations will shift from piloting AI technologies to making them operational by the end of 2024. Trying to scale AI across a big organization presents a new set of challenges.
“Getting one or two AI models into production is very different from running an entire enterprise or product on AI,” Vartak stated. Extreme intelligence can lead to extreme problems when things go wrong. One financial services company lost $20,000 in 10 minutes when a machine learning model began to drift, when properties of its target variable began to shift and the model got out of sync.
“With no visibility into the root issue — and no way to even identify which of its models was malfunctioning — the company was left with no choice but to pull the plug,” she stated. The new discipline of MLOps seeks to help organizations establish best practices and provide tools to support safe and efficient AI development, while working to operationalize AI systems. This requires investing in how things are done, in the people that do them, and in the tools they need.
To get there, AI teams first work to standardize how they build AI models and make them operational. Organizations need to have people in multiple roles work together to agree on a recommended way to build AI models, thus defining their own best practices.
Also, AI teams need to focus on what they are good at. “Building AI at scale can’t be produced by a single team — it requires a variety of unique skill sets, and very few individuals possess all of them,” Vartak stated. A data scientist can create a good algorithmic model. An ML engineer can integrate research models into products and monitor them. A single person is unlikely to be able to do both. Other roles include watching over governance, compliance and risk. As the AI scales, more expertise is required.
Business leaders should consider building specialized, dedicated AI teams that can focus on high-value strategic priorities, Vartak suggests. “Let data scientists do data science; let engineers do the engineering; let IT focus on infrastructure,” he stated.
She has seen two team models emerge. First is the “pod model,” where AI development is undertaken by a small team of a data scientist, data engineer and an ML or software engineer. The second is a “Center of Excellence” model, where the organization “pools” data science experts who are assigned to different product teams. “Both approaches have been implemented successfully and come with different pros and cons,” Vartak states.
An increasing number of deployed AI models in business will drive the need for discipline around the scaling of AI, predicts Kavita Ganesan, founder of Opinosis Analytics and author of the book, “The Business Case for AI.” As experience mounts from failed projects, AI teams are adjusting. “Data scientists are becoming more problem-focused,” Ganesan states on the company blog. “They’re adopting simpler techniques that will have a higher chance of success for real-world use cases. This change in thinking will improve the outcomes of many AI initiatives.”
Moreover, AI scientists and business leaders are having more discussions about the responsible use of AI and the social implications of AI projects.
“Informal conversations around AI ethics are just the beginning,” Ganesan states, adding, “Some of these discussions will turn into action where businesses will start having their own committees to vet AI systems rigorously. Some will even study potential societal impact before the release of specific technologies—regardless of regulations. Regulations will only add another layer of oversight, especially for companies that have yet to take AI ethics and accountability seriously.”
Industries that prefer to lag the bleeding edge such as hospitals, manufacturers and restaurant chains will also begin wider adoption of AI, as companies rethink their business models and adapt to a changed work world in the wake of the COVID-19 pandemic. “All options [will be] on the table for serious consideration,” she stated. “As part of this, AI will be a critical player in changing businesses forever.”
Read the source articles and information in VentureBeat, in Harvard Business Review and on the blog of Opinosis Analytics.
(Write to the editor here.)