Wiring ChatGPT for Business
Startups seek lanes for LLM-based businesses, as alternative AI platforms with predictable costs for training models emerge
By John P. Desmond, Editor, AI in Business
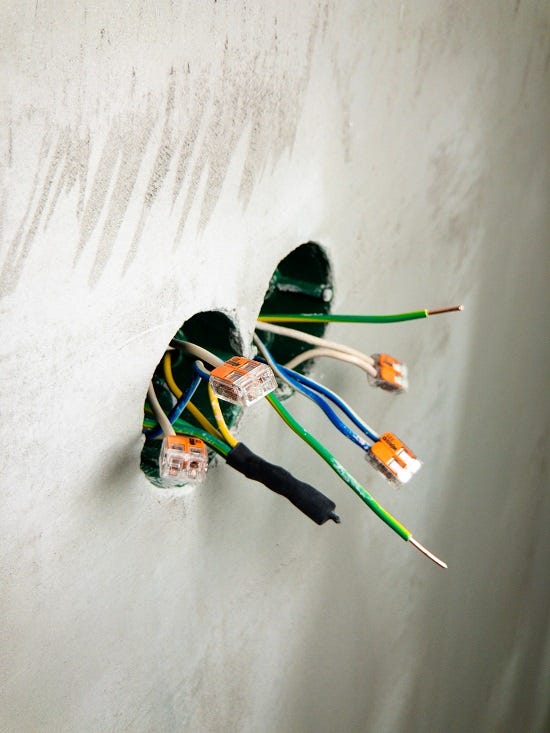
ChatGPT and other large language models take lots of computer processing power to run, processing that can run into some serious money, beyond the reach of many organizations.
And as large language model technology providers search for a business model, most likely in service and license fees, the industry is feeling ripple effects.
ChatGPT requires far more computing power to answer a question than Google uses to respond to a web search, according to a recent account from Bloomberg. “As organizations such as OpenAI seek to turn a profit, they may have to start charging for services that are now free,” stated Dina Bass, a technology reporter with Bloomberg News who authored the account.
Microsoft in January announced a $10 billion further investment in OpenAI, an agreement that gives OpenAI access to computing power on Azure, Microsoft’s cloud network. One observer characterized the arrangement as “cloud money laundering,” that disguises the true cost of subsidized computing power. “It creates a kind of unsustainable use case for machine learning,” stated Clement Delangue, CEO of Hugging Face, Inc., which houses a repository of open source AI models.
The cost of large language computing is related to the number of parameters the model supports; the more parameters, the higher the cost. ChatGPT’s model has 175 billion parameters. A popular model from Hugging Face has 10 billion parameters. Stable Diffusion from Stability AI, an alternative to the DALL-E image generator from OpenAI, has about one billion, according to the Bloomberg account. “There’s a trend this year that the models are getting bigger,” stated Tom Mason, CEO of Stability AI, noting that many engineers are at work to improve LLM efficiency.
OpenAI CEO Sam Altman has stated that the average cost per query to ChatGPT was probably single-digit cents per chat. Morgan Stanley estimated it to be two cents, which the firm said is seven times the average cost of a Google search query.
Some companies are seeking a lane to an LLM business model by focusing on a specific market. “One way for startups to go is to identify their area of specialization and focus their training models only on the relevant data,” stated Preeti Rathi, general partner at Icon Ventures Inc. in Palo Alto. Icon has invested in Aisera, which is working on a system to help resolve customer service tickets.
Other startups are starting out with general models from OpenAI or Stability AI, which they then customize with domain-specific data to target specific markets, stated Navrina Singh, CEO of startup Credo AI, which is working on supplying governance systems for new AI applications.
Google is reported to have had talks about investing $200 million in Cohere, an AI startup working on a language product for creating chatbots. Matt McIlwain, managing director at Madrona Venture Group LLC of Seattle, sees some shadow-boxing going on. “There’s somewhat of a proxy war going on between the big cloud companies,” he stated. “They are really the only ones that can afford to build the really big ones with gazillions of parameters.”
Venture capital firms have invested over $1.7 billion in generative AI solutions over the last three years, with AI-enabled drug discovery and software coding receiving the most funding, according to a recent report from Gartner.
“Early foundation models like ChatGPT focus on the ability of generative AI to augment creative work, but by 2025, we expect more than 30% — up from zero today — of new drugs and materials to be systematically discovered using generative AI techniques,” stated Brian Burke, Research VP for Technology Innovation at Gartner. “And that is just one of numerous industry use cases.”
While marketing and media businesses are among the first to feel the impact of ChatGPT and other generative AI models, Garter sees the following industries as posing good opportunities to apply generative AI:
Drug design, such as to bring down the cost of $1.8 billion average to bring a drug to market;
Material science, such as by a process called inverse design, in which the needed properties are defined up front and matched to materials that have those properties, reading potentially to entirely new materials;
Chip design, such as by speeding up the development life cycle;
Synthetic data, such as in healthcare where data can be artificially generated to conduct research and analysis; and
Design of parts, such as to meet specific goals and constraints, including a lighter design.
“The ability for technology to be creative is a game changer,” the Gartner authors stated.
Among the AI technology techniques employed by generative AI are foundation models, which the Gartner analysis describes as being pretrained on data sources in a self-supervised manner, which can then be adapted to solve new problems. Foundation models are based primarily on transformer architectures, which employ a deep neural network architecture.
Alternative Platforms for Training LLMs Emerging
An attempt to better quantify the cost to train large language models was recently attempted by the editors of The Next Platform, who examined a new system rental service for training GPT models available from Cerebras Systems, a machine learning system maker, and Cirrascale, a cloud computing company. “We now have some actual pricing that shows what it costs to run what GPT model at what scale,” stated Timothy Prickett Morgan, co-editor of The Next Platform and author of the account.
The pricing information is for doing GPT AI training on a quad of CS-2 supercomputers from Cerebras, working in partnership with Jasper, an AI application provider that is helping enterprises create a new way to deploy large language models to drive their applications. “Like just about everyone else on Earth, Jasper has been training its AI models on Nvidia GPUs, and it is looking for an easier and faster way to train models, which is how it makes a living,” stated Morgan.
Jasper’s customers are using its system to write blogs, create content marketing and generate technical manuals. While not perfect, they get to 70 percent of where they need to be, speeding up the process of content creation, according to Dave Rogenmoser, cofounder and CEO of Jasper, based in Austin
.“The enterprise businesses, first of all, want personalized models, and they want them badly,” Rogenmoser stated. “They want them trained in their language, they want them to be trained on their knowledge base and with their product catalogs. They want them trained on their brand voice – they want them to really be an extension of the brand.” And they want the models to help everyone in the company come up to speed quickly and speak the same language, and to self-optimize, continually get better and better.
Cerebras is offering its ‘Andromeda’ AI supercomputers, a set of 16 CS-2 wafer-scale systems lashed together into a single system that can deliver 120 petaflops of performance. The system costs just under $30 million, which makes the rental model attractive to startups.
“We believe that large language models are under-hyped and that we are just beginning to see the impact of them,” stated Andrew Feldman, cofounder and CEO of Cerebras, which is a pioneer in wafer-scale processing as well as an AI training hardware upstart. “There will be winners and new emergents in each of these three layers in the ecosystem –the hardware layer, and the infrastructure layer and foundation model, and in the application layer. And next year what you will see is the sweeping rise of and impact of large language models in various parts of the economy.”
The AI Model Studio from Cerebras and Cirrascale offers predictable costs.
“We have AI research labs and some financial institutions as customers, and all of them want to train their own models and use their own data to improve the accuracy of those models,” stated PJ Go, cofounder and CEO of Cirrascale. “And they want to do this at speed, at a reasonable price. And probably most importantly, they want a predictable price. They don’t want to write an open-ended blank check to a cloud service provider to be able to train a model.”
The Next Platform author anticipates that some companies will rent to train, and as they need to train more and larger models, “the economics will compel them to buy.”
Read the source articles and information from Bloomberg, from Gartner and from The Next Platform.
(Write to the editor here.)